Running out of time to catch up with new arXiv papers? We take the most impactful papers and present them as convenient podcasts. If you're a visual learner, we offer these papers in an engaging video format. Our service fills the gap between overly brief paper summaries and time-consuming full paper reads. You gain academic insights in a time-efficient, digestible format. Code behind this work: https://github.com/imelnyk/ArxivPapers Support this podcast: https://podcasters.spotify.com/pod/s ...
…
continue reading
This paper investigates how different prompt templates impact the performance of Large Language Models, revealing significant variations in effectiveness, particularly in code translation tasks. https://arxiv.org/abs//2411.10541 YouTube: https://www.youtube.com/@ArxivPapers TikTok: https://www.tiktok.com/@arxiv_papers Apple Podcasts: https://podcas…
…
continue reading
This paper investigates how different prompt templates impact the performance of Large Language Models, revealing significant variations in effectiveness, particularly in code translation tasks. https://arxiv.org/abs//2411.10541 YouTube: https://www.youtube.com/@ArxivPapers TikTok: https://www.tiktok.com/@arxiv_papers Apple Podcasts: https://podcas…
…
continue reading
The paper explores using sparse autoencoders to steer language model activations for safer responses, improving refusal behavior while noting potential negative impacts on overall performance. https://arxiv.org/abs//2411.11296 YouTube: https://www.youtube.com/@ArxivPapers TikTok: https://www.tiktok.com/@arxiv_papers Apple Podcasts: https://podcasts…
…
continue reading
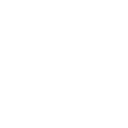
1
Steering Language Model Refusal with Sparse Autoencoders
24:02
24:02
Lire Plus Tard
Lire Plus Tard
Des listes
J'aime
Aimé
24:02
The paper explores using sparse autoencoders to steer language model activations for safer responses, improving refusal behavior while noting potential negative impacts on overall performance. https://arxiv.org/abs//2411.11296 YouTube: https://www.youtube.com/@ArxivPapers TikTok: https://www.tiktok.com/@arxiv_papers Apple Podcasts: https://podcasts…
…
continue reading
LLaVA-o1 is a novel Vision-Language Model that enhances reasoning in visual question-answering through structured multistage processes, outperforming larger models with fewer training samples. https://arxiv.org/abs//2411.10440 YouTube: https://www.youtube.com/@ArxivPapers TikTok: https://www.tiktok.com/@arxiv_papers Apple Podcasts: https://podcasts…
…
continue reading
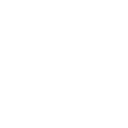
1
LLaVA-o1: Let Vision Language Models Reason Step-by-Step
17:55
17:55
Lire Plus Tard
Lire Plus Tard
Des listes
J'aime
Aimé
17:55
LLaVA-o1 is a novel Vision-Language Model that enhances reasoning in visual question-answering through structured multistage processes, outperforming larger models with fewer training samples. https://arxiv.org/abs//2411.10440 YouTube: https://www.youtube.com/@ArxivPapers TikTok: https://www.tiktok.com/@arxiv_papers Apple Podcasts: https://podcasts…
…
continue reading
The paper introduces affine concept editing (ACE) for controlling language model behavior through activation manipulation, demonstrating improved precision in managing refusal responses across various prompts. https://arxiv.org/abs//2411.09003 YouTube: https://www.youtube.com/@ArxivPapers TikTok: https://www.tiktok.com/@arxiv_papers Apple Podcasts:…
…
continue reading
The paper introduces affine concept editing (ACE) for controlling language model behavior through activation manipulation, demonstrating improved precision in managing refusal responses across various prompts. https://arxiv.org/abs//2411.09003 YouTube: https://www.youtube.com/@ArxivPapers TikTok: https://www.tiktok.com/@arxiv_papers Apple Podcasts:…
…
continue reading
The paper introduces Cut Cross-Entropy (CCE), a method that significantly reduces memory usage during training of large language models by optimizing cross-entropy loss computation without sacrificing performance. https://arxiv.org/abs//2411.09009 YouTube: https://www.youtube.com/@ArxivPapers TikTok: https://www.tiktok.com/@arxiv_papers Apple Podca…
…
continue reading
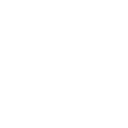
1
Cut Your Losses in Large-Vocabulary Language Models
17:27
17:27
Lire Plus Tard
Lire Plus Tard
Des listes
J'aime
Aimé
17:27
The paper introduces Cut Cross-Entropy (CCE), a method that significantly reduces memory usage during training of large language models by optimizing cross-entropy loss computation without sacrificing performance. https://arxiv.org/abs//2411.09009 YouTube: https://www.youtube.com/@ArxivPapers TikTok: https://www.tiktok.com/@arxiv_papers Apple Podca…
…
continue reading
Add-it is a training-free approach for semantic image editing that seamlessly integrates objects into images using a weighted extended-attention mechanism, achieving state-of-the-art results without fine-tuning. https://arxiv.org/abs//2411.07232 YouTube: https://www.youtube.com/@ArxivPapers TikTok: https://www.tiktok.com/@arxiv_papers Apple Podcast…
…
continue reading
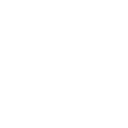
1
Add-it: Training-Free Object Insertion in Images With Pretrained Diffusion Models
19:23
19:23
Lire Plus Tard
Lire Plus Tard
Des listes
J'aime
Aimé
19:23
Add-it is a training-free approach for semantic image editing that seamlessly integrates objects into images using a weighted extended-attention mechanism, achieving state-of-the-art results without fine-tuning. https://arxiv.org/abs//2411.07232 YouTube: https://www.youtube.com/@ArxivPapers TikTok: https://www.tiktok.com/@arxiv_papers Apple Podcast…
…
continue reading
The SPA framework enhances user experience by generating diverse, high-quality responses from foundation models using synthetic data and data attribution methods, improving performance in code generation and natural language tasks. https://arxiv.org/abs//2411.06722 YouTube: https://www.youtube.com/@ArxivPapers TikTok: https://www.tiktok.com/@arxiv_…
…
continue reading
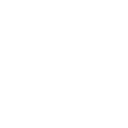
1
Synthesize, Partition, then Adapt: Eliciting Diverse Samples from Foundation Models
16:56
16:56
Lire Plus Tard
Lire Plus Tard
Des listes
J'aime
Aimé
16:56
The SPA framework enhances user experience by generating diverse, high-quality responses from foundation models using synthetic data and data attribution methods, improving performance in code generation and natural language tasks. https://arxiv.org/abs//2411.06722 YouTube: https://www.youtube.com/@ArxivPapers TikTok: https://www.tiktok.com/@arxiv_…
…
continue reading
…
continue reading
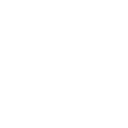
1
Aioli: A unified optimization framework for language model data mixing
29:42
29:42
Lire Plus Tard
Lire Plus Tard
Des listes
J'aime
Aimé
29:42
…
continue reading
This paper addresses imbalanced computation and memory in pipeline parallelism for large language models by partitioning vocabulary layers, reducing communication barriers, and achieving improved throughput and memory balance. https://arxiv.org/abs//2411.05288 YouTube: https://www.youtube.com/@ArxivPapers TikTok: https://www.tiktok.com/@arxiv_paper…
…
continue reading
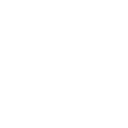
1
BALANCING PIPELINE PARALLELISM WITH VOCABULARY PARALLELISM
20:22
20:22
Lire Plus Tard
Lire Plus Tard
Des listes
J'aime
Aimé
20:22
This paper addresses imbalanced computation and memory in pipeline parallelism for large language models by partitioning vocabulary layers, reducing communication barriers, and achieving improved throughput and memory balance. https://arxiv.org/abs//2411.05288 YouTube: https://www.youtube.com/@ArxivPapers TikTok: https://www.tiktok.com/@arxiv_paper…
…
continue reading
This study explores whether pre-trained transformer models of chemical structures align with human olfactory perception, demonstrating their ability to predict expert labels and human ratings of odorants. https://arxiv.org/abs//2411.03038 YouTube: https://www.youtube.com/@ArxivPapers TikTok: https://www.tiktok.com/@arxiv_papers Apple Podcasts: http…
…
continue reading
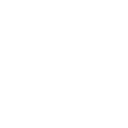
1
Can Transformers Smell Like Humans?
18:01
18:01
Lire Plus Tard
Lire Plus Tard
Des listes
J'aime
Aimé
18:01
This study explores whether pre-trained transformer models of chemical structures align with human olfactory perception, demonstrating their ability to predict expert labels and human ratings of odorants. https://arxiv.org/abs//2411.03038 YouTube: https://www.youtube.com/@ArxivPapers TikTok: https://www.tiktok.com/@arxiv_papers Apple Podcasts: http…
…
continue reading
The paper introduces Mixtures of In-Context Learners (MOICL), enhancing in-context learning by optimizing demonstration subsets, improving performance, and reducing memory usage in Transformer LLMs. https://arxiv.org/abs//2411.02830 YouTube: https://www.youtube.com/@ArxivPapers TikTok: https://www.tiktok.com/@arxiv_papers Apple Podcasts: https://po…
…
continue reading
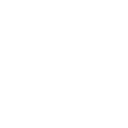
1
Mixtures of In-Context Learners
15:37
15:37
Lire Plus Tard
Lire Plus Tard
Des listes
J'aime
Aimé
15:37
The paper introduces Mixtures of In-Context Learners (MOICL), enhancing in-context learning by optimizing demonstration subsets, improving performance, and reducing memory usage in Transformer LLMs. https://arxiv.org/abs//2411.02830 YouTube: https://www.youtube.com/@ArxivPapers TikTok: https://www.tiktok.com/@arxiv_papers Apple Podcasts: https://po…
…
continue reading
OpenAI's Sora evaluates video generation models' ability to learn physical laws, revealing limitations in generalization and suggesting scaling alone isn't enough for uncovering fundamental principles. https://arxiv.org/abs//2411.02385 YouTube: https://www.youtube.com/@ArxivPapers TikTok: https://www.tiktok.com/@arxiv_papers Apple Podcasts: https:/…
…
continue reading
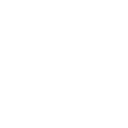
1
How Far Is Video Generation from World Model: A Physical Law Perspective
27:51
27:51
Lire Plus Tard
Lire Plus Tard
Des listes
J'aime
Aimé
27:51
OpenAI's Sora evaluates video generation models' ability to learn physical laws, revealing limitations in generalization and suggesting scaling alone isn't enough for uncovering fundamental principles. https://arxiv.org/abs//2411.02385 YouTube: https://www.youtube.com/@ArxivPapers TikTok: https://www.tiktok.com/@arxiv_papers Apple Podcasts: https:/…
…
continue reading
The paper introduces ADOPT, a new adaptive gradient method that resolves Adam's non-convergence issue without bounded noise assumptions, demonstrating superior performance across various deep learning tasks. https://arxiv.org/abs//2411.02853 YouTube: https://www.youtube.com/@ArxivPapers TikTok: https://www.tiktok.com/@arxiv_papers Apple Podcasts: h…
…
continue reading
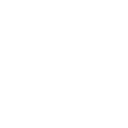
1
ADOPT: Modified Adam Can Converge with Any with the Optimal Rate
15:16
15:16
Lire Plus Tard
Lire Plus Tard
Des listes
J'aime
Aimé
15:16
The paper introduces ADOPT, a new adaptive gradient method that resolves Adam's non-convergence issue without bounded noise assumptions, demonstrating superior performance across various deep learning tasks. https://arxiv.org/abs//2411.02853 YouTube: https://www.youtube.com/@ArxivPapers TikTok: https://www.tiktok.com/@arxiv_papers Apple Podcasts: h…
…
continue reading
This study evaluates 17 leading Large Language Models' abilities in complex information retrieval, revealing many are thread-safe but have shorter effective context limits than supported lengths. https://arxiv.org/abs//2411.05000 YouTube: https://www.youtube.com/@ArxivPapers TikTok: https://www.tiktok.com/@arxiv_papers Apple Podcasts: https://podca…
…
continue reading
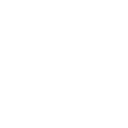
1
Needle Threading: Can LLMs Follow Threads through Near-Million-Scale Haystacks?
14:03
14:03
Lire Plus Tard
Lire Plus Tard
Des listes
J'aime
Aimé
14:03
This study evaluates 17 leading Large Language Models' abilities in complex information retrieval, revealing many are thread-safe but have shorter effective context limits than supported lengths. https://arxiv.org/abs//2411.05000 YouTube: https://www.youtube.com/@ArxivPapers TikTok: https://www.tiktok.com/@arxiv_papers Apple Podcasts: https://podca…
…
continue reading
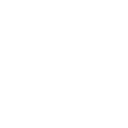
1
[QA] Mixture-of-Transformers: A Sparse and Scalable Architecture for Multi-Modal Foundation Models
7:53
https://arxiv.org/abs//2411.04996 YouTube: https://www.youtube.com/@ArxivPapers TikTok: https://www.tiktok.com/@arxiv_papers Apple Podcasts: https://podcasts.apple.com/us/podcast/arxiv-papers/id1692476016 Spotify: https://podcasters.spotify.com/pod/show/arxiv-papers --- Support this podcast: https://podcasters.spotify.com/pod/show/arxiv-papers/supp…
…
continue reading
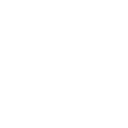
1
Mixture-of-Transformers: A Sparse and Scalable Architecture for Multi-Modal Foundation Models
41:18
41:18
Lire Plus Tard
Lire Plus Tard
Des listes
J'aime
Aimé
41:18
https://arxiv.org/abs//2411.04996 YouTube: https://www.youtube.com/@ArxivPapers TikTok: https://www.tiktok.com/@arxiv_papers Apple Podcasts: https://podcasts.apple.com/us/podcast/arxiv-papers/id1692476016 Spotify: https://podcasters.spotify.com/pod/show/arxiv-papers --- Support this podcast: https://podcasters.spotify.com/pod/show/arxiv-papers/supp…
…
continue reading
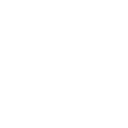
1
[QA] Do Mice Grok? Glimpses of Hidden Progress During Overtraining in Sensory Cortex
10:52
10:52
Lire Plus Tard
Lire Plus Tard
Des listes
J'aime
Aimé
10:52
The study reveals that task-specific representation learning continues in mice's piriform cortex during overtraining, enhancing classification accuracy despite behavior plateauing, suggesting hidden learning mechanisms at play. https://arxiv.org/abs//2411.03541 YouTube: https://www.youtube.com/@ArxivPapers TikTok: https://www.tiktok.com/@arxiv_pape…
…
continue reading
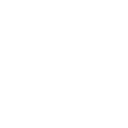
1
Do Mice Grok? Glimpses of Hidden Progress During Overtraining in Sensory Cortex
15:09
15:09
Lire Plus Tard
Lire Plus Tard
Des listes
J'aime
Aimé
15:09
The study reveals that task-specific representation learning continues in mice's piriform cortex during overtraining, enhancing classification accuracy despite behavior plateauing, suggesting hidden learning mechanisms at play. https://arxiv.org/abs//2411.03541 YouTube: https://www.youtube.com/@ArxivPapers TikTok: https://www.tiktok.com/@arxiv_pape…
…
continue reading
This study explores how transformers, both small and large, perform complex logical reasoning, identifying key circuits and mechanisms involved in planning and reasoning through a synthetic propositional logic problem. https://arxiv.org/abs//2411.04105 YouTube: https://www.youtube.com/@ArxivPapers TikTok: https://www.tiktok.com/@arxiv_papers Apple …
…
continue reading
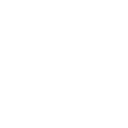
1
How Transformers Solve Propositional Logic Problems: A Mechanistic Analysis
22:34
22:34
Lire Plus Tard
Lire Plus Tard
Des listes
J'aime
Aimé
22:34
This study explores how transformers, both small and large, perform complex logical reasoning, identifying key circuits and mechanisms involved in planning and reasoning through a synthetic propositional logic problem. https://arxiv.org/abs//2411.04105 YouTube: https://www.youtube.com/@ArxivPapers TikTok: https://www.tiktok.com/@arxiv_papers Apple …
…
continue reading
We present a framework for end-to-end learning of data structures, optimizing query and space complexity, applied to nearest neighbor search and frequency estimation in data streams. https://arxiv.org/abs//2411.03253 YouTube: https://www.youtube.com/@ArxivPapers TikTok: https://www.tiktok.com/@arxiv_papers Apple Podcasts: https://podcasts.apple.com…
…
continue reading
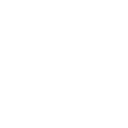
1
Discovering Data Structures: Nearest Neighbor Search and Beyond
28:18
28:18
Lire Plus Tard
Lire Plus Tard
Des listes
J'aime
Aimé
28:18
We present a framework for end-to-end learning of data structures, optimizing query and space complexity, applied to nearest neighbor search and frequency estimation in data streams. https://arxiv.org/abs//2411.03253 YouTube: https://www.youtube.com/@ArxivPapers TikTok: https://www.tiktok.com/@arxiv_papers Apple Podcasts: https://podcasts.apple.com…
…
continue reading
The paper examines factors influencing stimulus reconstruction fidelity, revealing that powerful generative models can mislead interpretations of neural signal extraction effectiveness. It proposes improved evaluation metrics for reconstruction methods. https://arxiv.org/abs//2411.02783 YouTube: https://www.youtube.com/@ArxivPapers TikTok: https://…
…
continue reading
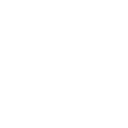
1
BrainBits: How Much of the Brain are Generative Reconstruction Methods Using?
15:29
15:29
Lire Plus Tard
Lire Plus Tard
Des listes
J'aime
Aimé
15:29
The paper examines factors influencing stimulus reconstruction fidelity, revealing that powerful generative models can mislead interpretations of neural signal extraction effectiveness. It proposes improved evaluation metrics for reconstruction methods. https://arxiv.org/abs//2411.02783 YouTube: https://www.youtube.com/@ArxivPapers TikTok: https://…
…
continue reading
Sparse Sinkhorn Token Translation (S2T2) improves text compression and inference in new domains by training tailored tokenizers and enabling effective token translation, enhancing performance in language models. https://arxiv.org/abs//2411.00593 YouTube: https://www.youtube.com/@ArxivPapers TikTok: https://www.tiktok.com/@arxiv_papers Apple Podcast…
…
continue reading
Sparse Sinkhorn Token Translation (S2T2) improves text compression and inference in new domains by training tailored tokenizers and enabling effective token translation, enhancing performance in language models. https://arxiv.org/abs//2411.00593 YouTube: https://www.youtube.com/@ArxivPapers TikTok: https://www.tiktok.com/@arxiv_papers Apple Podcast…
…
continue reading
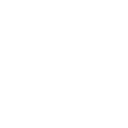
1
[QA] Decoding Dark Matter: Specialized Sparse Autoencoders for Interpreting Rare Concepts in Foundation Models
8:29
Specialized Sparse Autoencoders (SSAEs) enhance interpretability of foundation models by effectively capturing rare concepts, improving classification accuracy, and revealing insights into subdomain representations. https://arxiv.org/abs//2411.00743 YouTube: https://www.youtube.com/@ArxivPapers TikTok: https://www.tiktok.com/@arxiv_papers Apple Pod…
…
continue reading
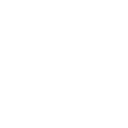
1
Decoding Dark Matter: Specialized Sparse Autoencoders for Interpreting Rare Concepts in Foundation Models
26:54
26:54
Lire Plus Tard
Lire Plus Tard
Des listes
J'aime
Aimé
26:54
Specialized Sparse Autoencoders (SSAEs) enhance interpretability of foundation models by effectively capturing rare concepts, improving classification accuracy, and revealing insights into subdomain representations. https://arxiv.org/abs//2411.00743 YouTube: https://www.youtube.com/@ArxivPapers TikTok: https://www.tiktok.com/@arxiv_papers Apple Pod…
…
continue reading
Tokenformer introduces a scalable architecture that enhances Transformers' efficiency by using token-parameter attention, allowing for incremental scaling without retraining, thus reducing computational costs significantly. https://arxiv.org/abs//2410.23168 YouTube: https://www.youtube.com/@ArxivPapers TikTok: https://www.tiktok.com/@arxiv_papers A…
…
continue reading
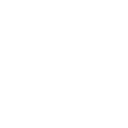
1
Tokenformer: Rethinking Transformer Scaling with Tokenized Model Parameters
19:10
19:10
Lire Plus Tard
Lire Plus Tard
Des listes
J'aime
Aimé
19:10
Tokenformer introduces a scalable architecture that enhances Transformers' efficiency by using token-parameter attention, allowing for incremental scaling without retraining, thus reducing computational costs significantly. https://arxiv.org/abs//2410.23168 YouTube: https://www.youtube.com/@ArxivPapers TikTok: https://www.tiktok.com/@arxiv_papers A…
…
continue reading
This paper challenges the assumption that academic researchers can't pre-train models, providing benchmarks and insights on optimizing GPU resources for efficient model training. https://arxiv.org/abs//2410.23261 YouTube: https://www.youtube.com/@ArxivPapers TikTok: https://www.tiktok.com/@arxiv_papers Apple Podcasts: https://podcasts.apple.com/us/…
…
continue reading
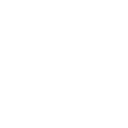
1
$100K or 100 Days: Trade-offs when Pre-Training with Academic Resources
16:51
16:51
Lire Plus Tard
Lire Plus Tard
Des listes
J'aime
Aimé
16:51
This paper challenges the assumption that academic researchers can't pre-train models, providing benchmarks and insights on optimizing GPU resources for efficient model training. https://arxiv.org/abs//2410.23261 YouTube: https://www.youtube.com/@ArxivPapers TikTok: https://www.tiktok.com/@arxiv_papers Apple Podcasts: https://podcasts.apple.com/us/…
…
continue reading
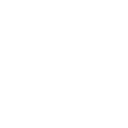
1
[QA] What Happened in LLMs Layers when Trained for Fast vs. Slow Thinking: A Gradient Perspective
7:59
This study analyzes layer-wise gradients in LLMs, revealing that slow thinking enhances learning stability and response correctness, while fast thinking shows larger gradient variations. https://arxiv.org/abs//2410.23743 YouTube: https://www.youtube.com/@ArxivPapers TikTok: https://www.tiktok.com/@arxiv_papers Apple Podcasts: https://podcasts.apple…
…
continue reading
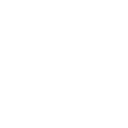
1
What Happened in LLMs Layers when Trained for Fast vs. Slow Thinking: A Gradient Perspective
15:27
15:27
Lire Plus Tard
Lire Plus Tard
Des listes
J'aime
Aimé
15:27
This study analyzes layer-wise gradients in LLMs, revealing that slow thinking enhances learning stability and response correctness, while fast thinking shows larger gradient variations. https://arxiv.org/abs//2410.23743 YouTube: https://www.youtube.com/@ArxivPapers TikTok: https://www.tiktok.com/@arxiv_papers Apple Podcasts: https://podcasts.apple…
…
continue reading
Tokenformer introduces a scalable architecture that enhances Transformers' efficiency by treating model parameters as tokens, allowing for flexible scaling without retraining, significantly reducing computational costs. https://arxiv.org/abs//2410.23168 YouTube: https://www.youtube.com/@ArxivPapers TikTok: https://www.tiktok.com/@arxiv_papers Apple…
…
continue reading
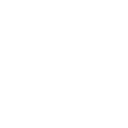
1
Tokenformer: Rethinking Transformer Scaling with Tokenized Model Parameters
19:38
19:38
Lire Plus Tard
Lire Plus Tard
Des listes
J'aime
Aimé
19:38
Tokenformer introduces a scalable architecture that enhances Transformers' efficiency by treating model parameters as tokens, allowing for flexible scaling without retraining, significantly reducing computational costs. https://arxiv.org/abs//2410.23168 YouTube: https://www.youtube.com/@ArxivPapers TikTok: https://www.tiktok.com/@arxiv_papers Apple…
…
continue reading